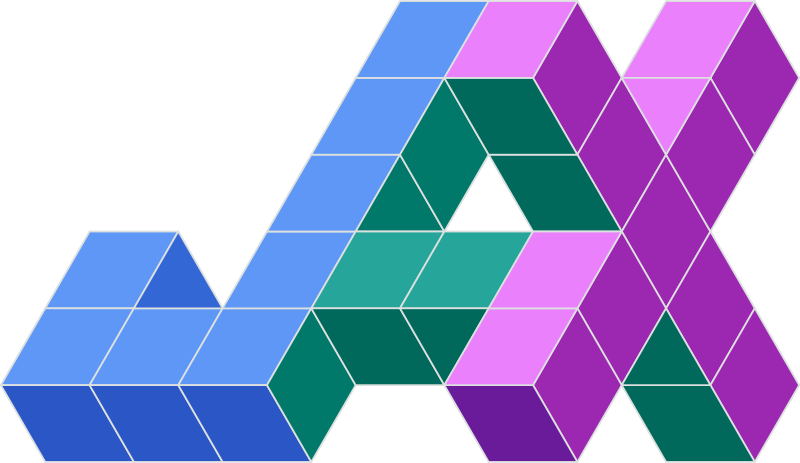
JAX 4 DC
JAX is an open-source system for high-performance numerical computing research. It offers the familiarity of Python+NumPy together with hardware acceleration, plus a set of function transformations that compose: automatic differentiation, compilation, batching, parallelization, and more.
Of particular interest to the L4DC community, JAX’s open-source community has developed extensible physics simulation libraries (e.g. Brax), trajectory optimizers (e.g. our own Trajax), and differential equation solvers (e.g. Diffrax). These compose neatly with JAX’s transformations, and with machine learning libraries in JAX’s ecosystem, enabling rapid experimentation at the interface of learning and control. For example, Trajax has served to power our research in high-speed ball-catching and learnable model-predictive control for human-friendly navigation.
This tutorial will start with JAX basics, demonstrate its use in combination with simulation and control libraries, and accelerate onward through state-of-the-art constrained trajectory optimizers (SQP).
Presenters: Roy Frostig (Google), Stephen Tu (Google), and Sumeet Singh (Google)
Location: Levine Hall, room 101 (Wu and Chen Auditorium)
Time: June 14, 2023, 09:00 – 12:15 (Coffee Break 10:15 – 10:45)
Schedule:
09:00 – 09:20 | Introduction to Jax, basic transformations |
09:20 – 10:15 | Jax as a building block for optimal control:first order methods, iLQR, vectorization of solvers, automatic differentiation of solutions |
10:15 – 10:45 | Coffee Break |
10:45 – 12:15 | Fundamentals of trajectory optimization, including iLQR and SQP solvers for constrained optimization |
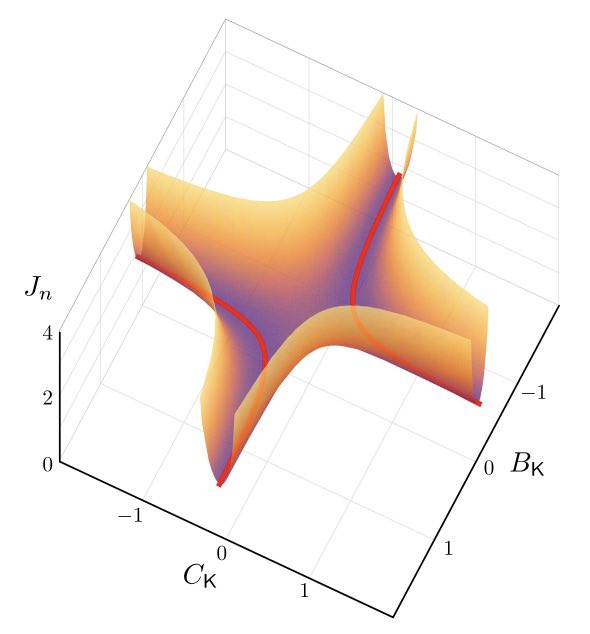
Toward a Theoretical Foundation of Policy Optimization for Learning Control Policies
Gradient-based methods have been widely used for system design and optimization in diverse application domains. This tutorial surveys some of the recent developments on policy optimization, a gradient-based iterative approach for feedback control synthesis, popularized by successes of reinforcement learning. The presenters will take an interdisciplinary perspective in their exposition that connects control theory, reinforcement learning, and large-scale optimization. Further, a number of recently-developed theoretical results on the optimization landscape, global convergence, and sample complexity of gradient-based methods for various continuous control problems will be discussed.
Presenters: Maryam Fazel (UW), Bin Hu (UIUC), Na Li (Harvard), Kaiqing Zhang (Maryland)
Location: Levine Hall, room 101 (Wu and Chen Auditorium)
Time: June 14, 2023, 14:00 – 17:15
Slides: download
Schedule:
14:00 – 14:30 | Opening, brief history, a new look from RL, optimization basics |
14:30 – 15:00 | Policy Optimization Theory for LQR |
15:00 – 15:30 | Mixed design and risk sensitive control, LQ games |
15:30 – 16:00 | Coffee Break |
16:00 – 16:30 | Policy search for H-infinity control: nonsmooth optimization |
16:30 – 17:00 | LQG landscape and analysis |
17:00 – 17:15 | Convex parameterization |
17:15 – 17:30 | Future work and discussion |