The keynote talks will take place in the Irvine Auditorium.
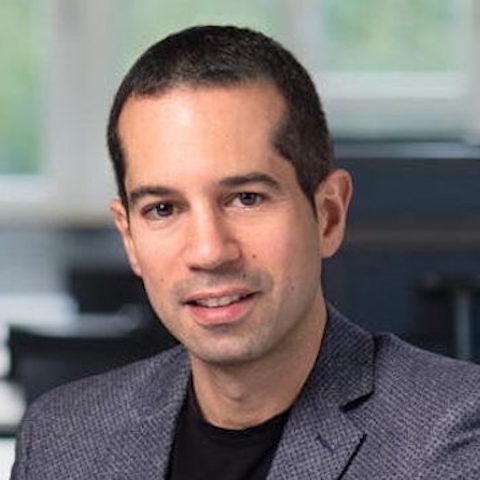
Davide Scaramuzza (UZH)
Human-Level Performance with Autonomous Vision-based Drones
(Thursday, June 15, 09:00 – 09:45)
Abstract: Autonomous drones play a crucial role in search-and-rescue, delivery, and inspection missions, and promise to increase productivity by a factor of 10. However, they are still far from human pilots regarding speed, versatility, and robustness. What does it take to fly autonomous drones as agile as or even better than human pilots? Autonomous, agile navigation through unknown, GPS-denied environments poses several challenges for robotics research in terms of perception, learning, planning, and control. In this talk, I will show how the combination of both model-based and machine learning methods united with the power of new, low-latency sensors, such as event cameras, can allow drones to achieve unprecedented speed and robustness by relying solely on onboard computing. This can result in better productivity and safety of future autonomous aircraft.
Bio: Davide Scaramuzza is a Professor of Robotics and Perception at the University of Zurich, where he does research at the intersection of robotics, computer vision, and machine learning. He did his PhD at ETH Zurich, a postdoc at the University of Pennsylvania, and was visiting professor at Stanford University. His research focuses on autonomous, agile navigation of micro drones using both standard and neuromorphic event-based cameras. He pioneered autonomous, vision-based navigation of drones, which inspired the navigation algorithm of the NASA Mars helicopter. He has been serving as a consultant for the United Nations on topics such as disaster response and disarmament, as well as the Fukushima Action Plan on Nuclear Safety. He won many prestigious awards, such as a European-Research-Council Consolidator grant, the IEEE Robotics and Automation Society Early Career Award, an SNF-ERC Starting Grant, a Google Research Award, a Facebook Distinguished Faculty Research Award, two NASA TechBrief Awards, and many paper awards (TRO, RSS, IROS, CORL, CVPR, etc.). In 2015, he co-founded Zurich-Eye, today Facebook Zurich, which developed the world-leading virtual-reality headset, Oculus Quest, which sold over 10 million units. In 2020, he co-founded SUIND, which builds autonomous drones for precision agriculture. Many aspects of his research have been prominently featured in broader media, such as The New York Times, The Economist, Forbes, BBC News, and Discovery Channel.
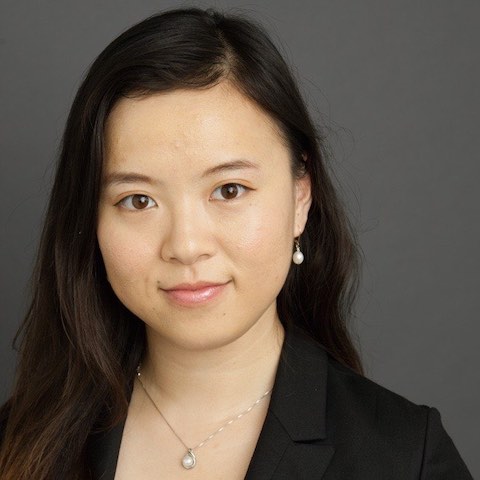
Rose Yu (UC San Diego)
On the Interplay Between Deep Learning and Dynamical Systems
(Thursday, June 15, 11:15 – 12:00)
Abstract: The explosion of spatiotemporal data in the physical world requires new deep learning tools to model complex dynamical systems. On the other hand, dynamical system theory plays a key role in understanding the emerging behavior of deep neural networks. In this talk, I will give an overview of our research to explore the interplay between the two. I will showcase the applications of these approaches in fluid mechanics, autonomous driving, and optimization.
Bio: Dr. Rose Yu is an assistant professor at the University of California San Diego, Department of Computer Science and Engineering. She earned her Ph.D. in Computer Sciences at USC in 2017. She was subsequently a Postdoctoral Fellow at Caltech. Her research focuses on advancing machine learning techniques for large-scale spatiotemporal data analysis, with applications to sustainability, health, and physical sciences. A particular emphasis of her research is on physics-guided AI which aims to integrate first principles with data-driven models. Among her awards, she has won Army ECASE Award, NSF CAREER Award, Hellman Fellow, Faculty Research Award from JP Morgan, Facebook, Google, Amazon, and Adobe, Several Best Paper Awards, Best Dissertation Award at USC, and was nominated as one of the ’MIT Rising Stars in EECS’.
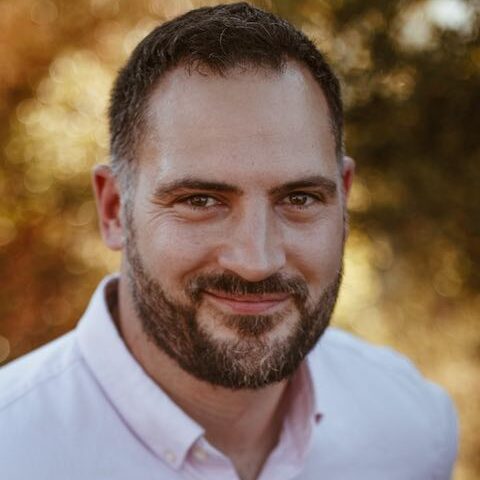
Aaron Ames (Caltech)
Safety in Theory and Practice: Why Learning Needs Control
(Thursday, June 15, 14:00 – 14:45)
Abstract: As robotic systems pervade our everyday lives, especially those that leverage complex learning and autonomy algorithms, the question becomes: how can we trust that robots will operate safely around us? An answer to this question was given, in the abstract, by famed science fiction writer Isaac Asimov: the three laws of robotics. These three laws—that a robot (1) may not harm a human, (2) must do what it is ordered, and (3) cannot harm itself—provide a safety layer between the robot and the world that ensures its safe behavior. In this presentation I will propose a mathematical formalization of the three laws of robots, encapsulated by control barrier functions (CBFs). These generalizations of (control) Lyapunov functions ensure forward invariance of “safe” sets. Moreover, CBFs lead to the notion of a safety filter that minimally modifies an existing controller to ensure safety of the system—even if this controller is unknown, the result of a learning-based process, or operating as part of a broader layered autonomy stack. The utility of CBFs will be demonstrated through their extensive implementation in practice on a wide variety of highly dynamic robotic systems: from ground robots, to drones, to legged robots, to robotic assistive devices.
Bio: Aaron D. Ames is the Bren Professor of Mechanical and Civil Engineering and Control and Dynamical Systems at the California Institute of Technology (Caltech). He received a B.S. in Mechanical Engineering and a B.A. in Mathematics from the University of St. Thomas in 2001 and received a M.A. in Mathematics and a Ph.D. in Electrical Engineering and Computer Sciences from UC Berkeley in 2006. He served as a Postdoctoral Scholar in Control and Dynamical Systems at Caltech from 2006 to 2008, began his faculty career at Texas A&M University in 2008, and was an Associate Professor in Mechanical Engineering and Electrical & Computer Engineering at the Georgia Institute of Technology before joining Caltech in 2017. He is an IEEE Fellow and has received multiple awards for his research in control, including: the NSF CAREER award in 2010, the 2015 Donald P. Eckman Award, and the 2019 Antonio Ruberti Young Researcher Prize. His research interests span the areas of nonlinear control, safety-critical, cyber-physical and hybrid systems, with a special focus on applications to robotic systems—both formally and through experimental validation. The application of these ideas ranges from enabling autonomy in robotic systems while ensuring safety, to improving the locomotion capabilities of the mobility impaired. The publications produced by his lab have received numerous best paper awards at top conferences on robotics and control.
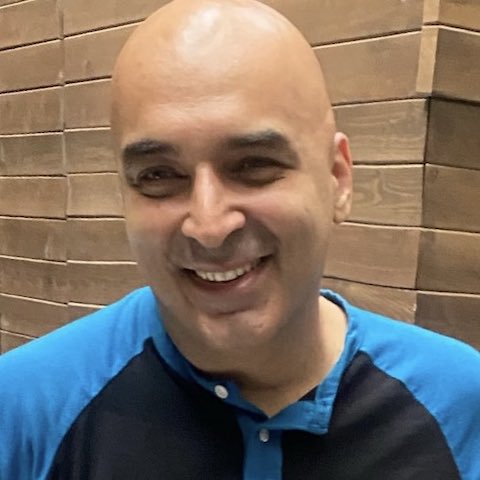
Vikas Sindhwani (Google Brain)
Large Language Models with Eyes, Arms and Legs
(Friday, June 16, 09:00 – 09:45)
Abstract: To become useful in human-centric environments, robots must demonstrate language comprehension, semantic understanding and logical reasoning capabilities working in concert with low-level physical skills. With the advent of modern “foundation models” trained on massive datasets, the algorithmic path to developing general-purpose “robot brains” is (arguably) becoming clearer, though many challenges remain. In the first part of this talk, I will attempt to give a flavor of how state-of-the-art multimodal foundation models are built, and how they can be bridged with low-level control. In the second part of the talk, I will summarize a few surprising lessons on control synthesis observed while solving a collection of Robotics benchmarks at Google. I will end with some emerging open problems and opportunities at the intersection of dynamics, control and foundation models.
Bio: Vikas Sindhwani is Research Scientist at Google Deepmind in New York where he leads a research group focused on solving a range of planning, perception, learning, and control problems arising in Robotics. His interests are broadly in core mathematical foundations of statistical machine learning, and in end-to-end design aspects of building large-scale and robust AI systems. He received the best paper award at Uncertainty in Artificial Intelligence (UAI-2013), the IBM Pat Goldberg Memorial Award in 2014, and was finalist for Outstanding Planning Paper Award at ICRA-2022. He serves on the editorial board of Transactions on Machine Learning Research (TMLR) and IEEE Transactions on Pattern Analysis and Machine Intelligence; he has been area chair and senior program committee member for NeurIPS, International Conference on Learning Representations (ICLR) and Knowledge Discovery and Data Mining (KDD). He previously headed the Machine Learning group at IBM Research, NY. He has a PhD in Computer Science from the University of Chicago and a B.Tech in Engineering Physics from Indian Institute of Technology (IIT) Mumbai. His publications are available at: http://vikas.sindhwani.org/
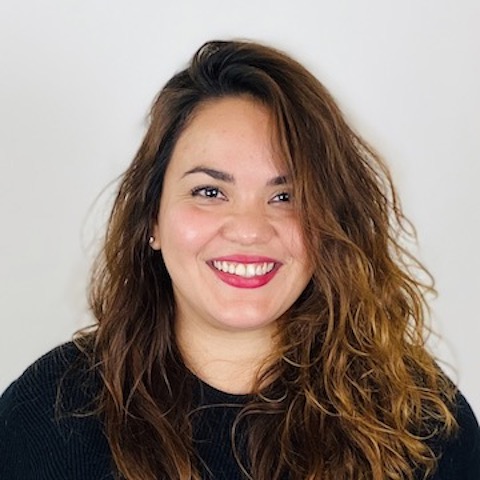
Nadia Figueroa (Penn)
Safety, Adaptation and Efficient Learning in Physical Human-Robot Interaction: A Dynamical Systems Approach
(Friday, June 16, 11:15 – 12:00)
Abstract: For the last decades we have lived with the promise of one day being able to own a robot that can coexist, collaborate and cooperate with humans in our everyday lives. This has motivated a vast amount of research on robot control, motion planning, machine learning, perception and physical human-robot interaction (pHRI). However, we are yet to see robots fluidly collaborating with humans and other robots in the human-centric dynamic spaces we inhabit. This deployment bottleneck is due to traditionalist views of how robot tasks and behaviors should be specified and controlled. For collaborative robots to be truly adopted in such dynamic, ever-changing environments they must be adaptive, compliant, reactive, safe and easy to teach or program. Combining these objectives is challenging as providing a single optimal solution can be intractable and even infeasible due to problem complexity, time-critical and safety-critical requirements and contradicting goals. In this talk, I will show that with a Dynamical Systems (DS) approach for motion planning and pHRI we can achieve reactive, provably safe and stable robot behaviors while efficiently teaching the robot complex tasks with a handful of demonstrations. Such an approach can be extended to offer task-level reactivity, transferability and can be used to incrementally learn from new data and failures in a matter of seconds, just as humans do. I will also discuss the role of compliance in collaborative robots, the allowance of soft impacts and the relaxation to the standard definition of safety in pHRI and how this can be achieved with DS-based and optimization-based approaches. Finally, I will talk about the importance of efficient and geometrically rigorous safety boundary representations and present learning-based and sample-based techniques that can achieve real-time safety guarantees in task-space and joint-space for multi-DoF robots interacting with humans.
Bio: Nadia Figueroa is the Shalini and Rajeev Misra Presidential Assistant Professor in the Mechanical Engineering and Applied Mechanics Department at the University of Pennsylvania. She holds secondary appointments in Computer and Information Science and Electrical and Systems Engineering and is a member of the GRASP laboratory. She received a B.Sc. degree in Mechatronics from the Monterrey Institute of Technology, Mexico in 2007, an M.Sc. degree in Automation and Robotics from the Technical University of Dortmund, Germany in 2012 and a Ph.D. in Robotics, Control and Intelligent Systems at the Swiss Federal Institute of Technology in Lausanne, Switzerland (EPFL) in 2019. Prior to joining Penn, she was a Postdoctoral Associate in the Interactive Robotics Group of the Computer Science and Artificial Intelligence Laboratory (CSAIL) at the Massachusetts Institute of Technology from 2020 to 2022. Her research focuses on developing control and learning algorithms for collaborative human-aware robotic systems: robots that can safely and efficiently interact with humans and other robots in the human-centric dynamic spaces we inhabit. Her Ph.D thesis was a finalist for the Georges Giralt Ph.D. award in 2020 – the best European Ph.D. thesis in robotics, the ABB PhD Award and the EPFL Doctoral Distinction Award. Her co-authored work on multi-robot human collaboration was a finalist for the KUKA Innovation Award in 2017, Best Systems and Best Conference Paper Award and winner of the Best Student Paper Award at the 2016 Robotics: Science and Systems (RSS) Conference.
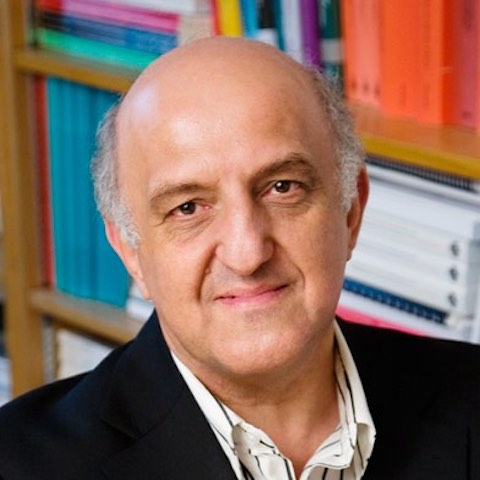
Tamer Basar (UIUC)
Consensus and Dissensus in Multi-agent Dynamical Systems with Learning
(Friday, June 16, 14:00 – 14:45)
Abstract: Perhaps the most challenging aspect of research on multi-agent dynamical systems, naturally formulated as non-cooperative stochastic differential/dynamic games (SDGs) with asymmetric dynamic information structures, is the presence of strategic interactions among agents, with each one developing beliefs on others in the absence of shared information. This belief generation process involves what is known as a second-guessing phenomenon, which generally entails infinite recursions, thus compounding the difficulty of obtaining (and arriving at) an equilibrium. This difficulty is somewhat alleviated when there is a high population of agents (players), in which case strategic interactions at the level of each agent become much less pronounced. With some structural specifications, this leads to what is known as mean field games (MFGs), which have been the subject of intense research activity during the last fifteen years or so.
This talk will first provide a general overview of fundamentals of MFGs approach to decision making in multi-agent dynamical systems in both model-based and model-free settings, and discuss connections to finite-population games. Following this general introduction, the talk will focus, for concrete results, on the structured setting of discrete-time infinite-horizon linear-quadratic-Gaussian dynamic games, where the players are partitioned into finitely-many populations with an underlying graph topology—a framework motivated by paradigms where consensus and dissensus co-exist. MFGs approach will be employed to arrive at approximate Nash equilibria, with a precise quantification of the approximation as a function of population sizes. For the model-free versions of such games, a reinforcement learning algorithm will be introduced based on zero-order stochastic optimization, along with guaranteed convergence. The talk will also address derivation of a finite-sample bound, quantifying the estimation error as a function of the number of samples, and will conclude with a discussion of some extensions of the general setting and future research directions.
Bio: Tamer Başar has been with University of Illinois Urbana-Champaign since 1981, where he is currently Swanlund Endowed Chair Emeritus; CAS Professor Emeritus of ECE; and Research Professor, CSL and ITI. He has served as Director of the Center for Advanced Study (2014-2020), Interim Dean of Engineering (2018), and Interim Director of the Beckman Institute (2008-2010). He is a member of the US National Academy of Engineering and the American Academy of Arts and Sciences; Fellow of IEEE, IFAC, and SIAM; and has served as presidents of the IEEE Control Systems Society (CSS), the International Society of Dynamic Games (ISDG), and the American Automatic Control Council (AACC). He has received several awards and recognitions over the years, including the highest awards of IEEE CSS, IFAC, AACC, and ISDG, the IEEE Control Systems Technical Field Award, Wilbur Cross Medal from his alma mater Yale, and a number of international honorary doctorates and professorships. He was Editor-in-Chief of the IFAC Journal Automatica between 2004 and 2014, and is currently editor of several book series. He has contributed profusely to fields of systems, control, communications, optimization, networks, and dynamic games, and has current research interests in stochastic teams, games, and networks; multi-agent systems and learning; data-driven distributed optimization; epidemics modeling and control over networks; strategic information transmission, spread of disinformation, and deception; security and trust; energy systems; and cyber-physical systems.